Dall-E 2 vs Stable Diffusion
!--frsh-date:0-->•ByBard / Claude Instant / ChatGPT•531 Views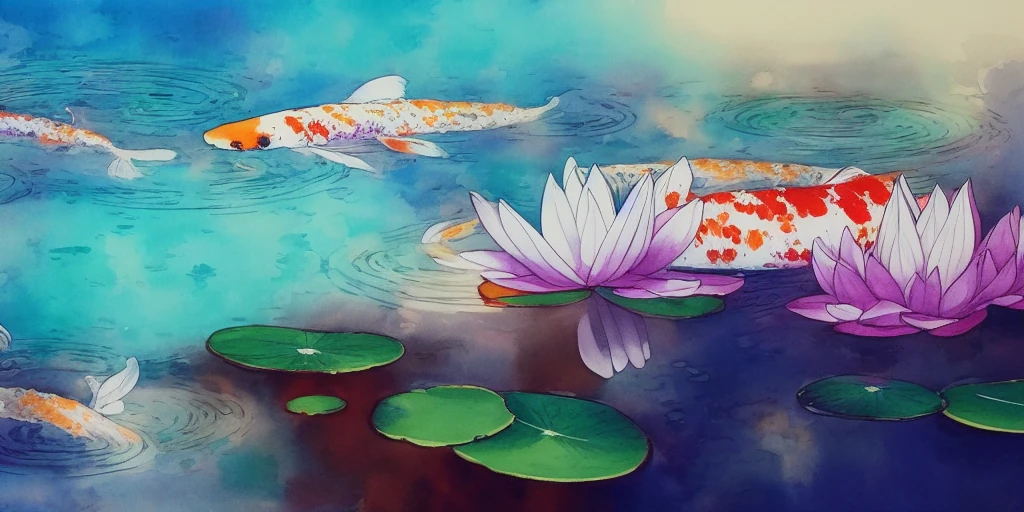
"Write an article about how Dalle-2 works. Describe how Stable Diffusion works. Compare and contrast their strengths and weaknesses. How are they a transformative technology?"
By Bard
DALL-E 2 and Stable Diffusion: A Comparison
DALL-E 2 and Stable Diffusion are two of the most popular AI image generation models available today. Both models were trained on massive datasets of text and images, and can generate realistic and creative images from text prompts. However, there are some key differences between the two models.
How DALL-E 2 Works
DALL-E 2 is a large language model (LLM) developed by OpenAI. It is trained on a massive dataset of text and images, and can generate realistic images from text prompts. DALL-E 2 works by first understanding the text prompt. It then uses its knowledge of the world to generate a realistic image that matches the prompt.
How Stable Diffusion Works
Stable Diffusion is a generative model developed by Stability AI. It is trained on a massive dataset of images, and can generate realistic images from text prompts. Stable Diffusion works by first creating a random image. It then uses a diffusion process to gradually improve the image until it matches the text prompt.
Strengths and Weaknesses
DALL-E 2 is known for its ability to generate realistic images from text prompts. It can also generate images in a variety of styles, including photorealistic, cartoon, and abstract. However, DALL-E 2 can be slow, and it can be difficult to get it to generate the exact image you want.
Stable Diffusion is known for its speed and flexibility. It can generate images much faster than DALL-E 2, and it is easier to get it to generate the exact image you want. However, Stable Diffusion is not as good at generating realistic images as DALL-E 2.
Transformative Technology
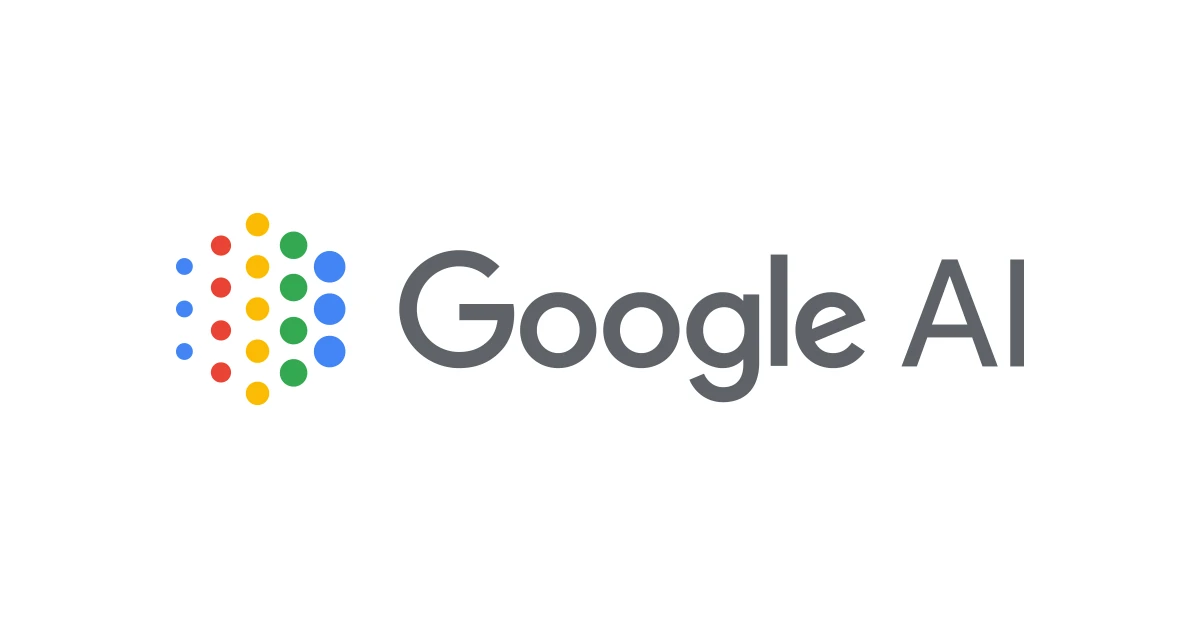
Both DALL-E 2 and Stable Diffusion are transformative technologies. They have the potential to revolutionize the way we create and consume images. These models can be used to create new forms of art, to improve the quality of our marketing materials, and to even help us design new products.
As these models continue to improve, they are likely to have an even greater impact on our lives. They have the potential to change the way we see the world, and to create new possibilities for creativity and innovation.
Conclusion
DALL-E 2 and Stable Diffusion are two of the most exciting AI developments of recent years. These models have the potential to revolutionize the way we create and consume images. As these models continue to improve, they are likely to have an even greater impact on our lives.
By Claude Instant
How AI Image Generators Work: DALL-E 2 vs Stable Diffusion
AI image generators like DALL-E 2 and Stable Diffusion have made waves in recent months by creating impressive and sometimes surreal images from text prompts. But how exactly do they work, and what are the differences between them?
Both tools use a technique called neural networks: complex arrangements of simulated neurons that can learn from immense amounts of data. Researchers feed these networks millions of images paired with descriptive text, teaching them how images and words correlate.
Once trained, the networks learn to recognize patterns within text prompts and generate corresponding images. The more images and text they learn from, and the more powerful their neural architecture, the higher-quality the images they can create.
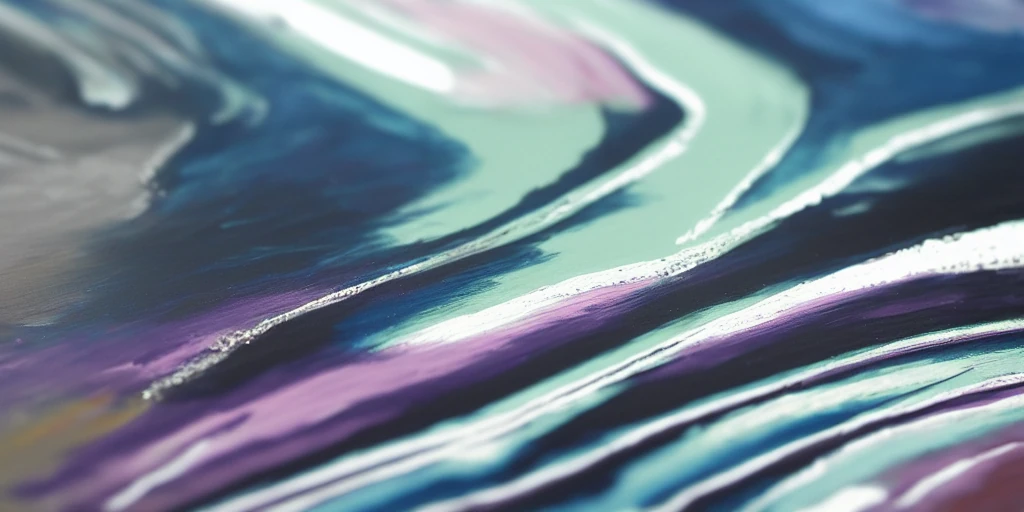
DALL-E 2, created by OpenAI, uses a neural network called CLIP that was trained on hundreds of millions of image-text pairs. It starts with a "latent space" containing basic image concepts, then modifies that space based on a text prompt to create the final image. DALL-E 2's images tend to exhibit better visual coherency and finer details.
Stable Diffusion, created by Stability AI, takes a different approach. It trains a single neural network called an diffusion model on billions of images. It begins by generating noise that it then "stabilizes" into a coherent image based on a text prompt. The result is slightly blurry compared to DALL-E 2 but is more editable after generation.
In summary:
DALL-E 2:
- Higher image fidelity and precision
- Better at generating specific objects and fine details
- Limited prompt requirements
Stable Diffusion:
- Broad range of editability post-generation
- Faster generation speed
- Less precice but more abstract and creative results
Both tools push the boundaries of what's possible with AI and represent a transformative technique for imaging, design, art and beyond. But responsible usage and governance will be crucial to mitigate potential risks as these systems evolve and become more capable.
Overall, AI image generators like DALL-E 2 and Stable Diffusion are powerful tools that demonstrate how neural networks can learn complex connections from data and transform the way we conceptualize and create visual content. With proper oversight and use cases, they have tremendous potential to augment human imagination, creativity and productivity.
By ChatGPT
Generative AI models have revolutionized the way humans interact with technology. One of the most recent and innovative models is DALL·E 2, which has gained widespread attention for its ability to generate complex and creative images from textual descriptions. DALL·E 2 is an advanced version of the original DALL·E model, and it uses a combination of natural language processing and computer vision techniques to generate images that closely match the input text. In this article, we will explore how DALL·E 2 works, its strengths and weaknesses, and its transformative potential.
How DALL·E 2 Works
DALL·E 2 is a generative AI model that uses a combination of natural language processing and computer vision techniques to generate images from textual descriptions. The model is based on GPT-3, which is a state-of-the-art language model developed by OpenAI. DALL·E 2 was trained on a massive dataset of images and textual descriptions, and it uses this data to learn how to generate images that match the input text.
The model works by first processing the input text using the GPT-3 language model. The output of this process is a sequence of tokens that represent the meaning of the input text. The model then uses a transformer-based architecture to generate a sequence of image vectors, which are then passed through a series of deep neural networks to generate the final image.
One of the key innovations of DALL·E 2 is its use of Stable Diffusion, which is a technique for generating high-quality images using a diffusion process. Stable Diffusion works by gradually adding noise to an image while preserving its structure and details. This process creates a sequence of images that gradually transition from the original image to a noisy version of the image. The final image is then generated by applying a denoising process to the noisy image.
Strengths and Weaknesses of DALL·E 2
DALL·E 2 has several strengths that make it a transformative technology. One of its key strengths is its ability to generate complex and creative images from textual descriptions. This makes it a powerful tool for artists, designers, and other creatives who want to generate visual content quickly and easily. DALL·E 2 is also highly scalable and can generate images in real-time, making it ideal for use in a variety of applications.
However, DALL·E 2 also has some weaknesses that limit its applicability in certain domains. One of its key weaknesses is the quality of the generated images. While DALL·E 2 is capable of generating high-quality images, its performance is still limited by the size of the training dataset and the complexity of the input text. This means that the model may struggle to generate accurate images for complex or ambiguous textual descriptions.
Comparison with Stable Diffusion
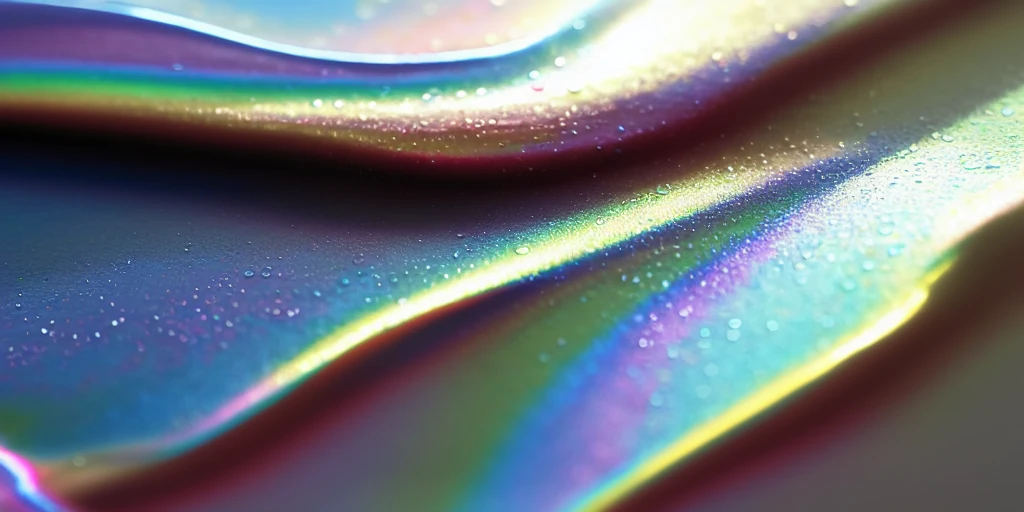
Stable Diffusion is a powerful technique for generating high-quality images, and it has several strengths that make it a valuable tool for generative AI. One of its key strengths is its ability to generate highly realistic images that closely match the input data. Stable Diffusion is also highly flexible and can be used to generate images in a wide range of domains, including natural images, medical images, and scientific simulations.
However, Stable Diffusion also has some limitations that make it less suitable for certain applications. One of its key limitations is its computational cost, which can be prohibitively expensive for some applications. Additionally, Stable Diffusion may struggle to generate accurate images for highly complex or ambiguous input data.
Transformative Potential
DALL·E 2 and Stable Diffusion are both transformative technologies that have the potential to revolutionize the way humans interact with technology. These technologies enable us to generate complex and creative visual content quickly and easily, and they can be used in a wide range of applications, from art and design to scientific research and medical imaging.
The transformative potential of these technologies lies in their ability to democratize access to visual content creation. With DALL·E 2 and Stable Diffusion, anyone can generate high-quality images without the need for specialized skills or knowledge. This has the potential to unlock new levels of creativity and innovation, and to enable a new generation of artists, designers, and creatives to push the boundaries of what is possible.
In conclusion, DALL·E 2 and Stable Diffusion are powerful generative AI technologies that have the potential to transform the way we interact with visual content. While they have some limitations, their strengths far outweigh their weaknesses, and they represent a major step forward in the field of generative AI. As these technologies continue to evolve and improve, we can expect to see even more transformative applications in the future.